The A-PR Hypothesis focuses on two key capacities of life, which together make evolution toward increased functionality possible: autonomy of individuals as unique
participants in their ecosystems and their differing capacities for pattern recognition.
|
|

In her book If Microbes begat Mind: from origins of life to emergence of intelligence Zann Gill postulates the A-PR Hypothesis to address five intersecting challenges:
• contradictions in definitions of life;
• debates about the origins of life;
• arguments about the source of evolutionary novelty and direction of evolution’s arrow;
• questions raised about technology’s aim to build intelligent, self-improving systems, and
• the need for next generation social networks to evolve into problem-solving ecosystems.
Intelligent systems modeled on life are informed by parallels between LUCA (our Last Universal Common Ancestor) and distributed knowledge networks. Hypothesis formation about origins sheds light on our problem-solving methods. The A-PR Hypothesis (Autonomy and Pattern Recognition) characterizes how a web-like view of LUCA, as life’s original, open source, cross-platform software coding experiment, can inform our efforts to create future systems manifesting collaborative intelligence.
|
 |
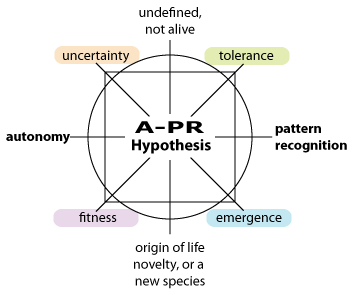
    
© Zann Gill 2011
|
The diagram left postulates the structure for a single A-PR cycle of a self-improving system through which what is not alive becomes alive.
Autonomy. Individual contributors collaborate, maintaining their diversity of roles and priorities as they apply unique skills in a problem-solving process. Individuals are not homogenized, as in consensus-driven processes, nor equalized through quantitative data processing, as in collective intelligence.
Pattern Recognition. Unique capacities for pattern recognition and interpretation characterize living systems and enable them to choose appropriate (or not) actions in context, driving evolution toward increased functional effectiveness.
|
The A-PR capacity occurs in iterative learning, self-improving systems. Artificial systems, to mimic life's capacity to discriminate on its own behalf, must be able to bootstrap themselves toward improved functionality by their choices. The starting condition is necessarily characterized by its uncertainty. Across the range of necessary prerequisite conditions tolerance spectra, when overlaid, must allow a “window of opportunity” for the emergence of a life-like system, whose fitness is tested in context. Selection for fitness computes the integrated synergy of the interractions of the living organism (its smart, appropriate choices) with its environment, a persistent recycling into the uncertainty of the future. And so the cycle repeats, bootstrapping life and intelligence as a single symbiotic system.
|
|
 |
  |
The peacock’s tail is a classic instance where pattern recognition enables choice in evolution. An indifferent environment would be unlikely to select peacock tails as the most efficient survival mechanism. As lady peahens choose peacocks with grander, more unwieldy, flamboyant tails, the tails themselves do not make those peacocks more fit.
The bird who can wield his unwieldy tail, and still survive, has made a show of fitness. The peacock’s tail is large and blows around in the wind. Its bright colors attract predators. The peacock would probably survive better without his tail. The peacock’s tail evolved, guided by members of its species. |
|
When a peahen chooses her partner for his magnificent tail, her pattern recognition and choice drive evolution. Good genes, the traditional view, versus good taste sum up two opposing views.While we easily accept the role of pattern recognition in the evolution of a peacock’s tail, as peahens assess the tails of their peacock suitors, the assumption that pattern recognition is a fundamental defining attribute of life, even at the molecular level is less obvious. An internet search of the key words “molecular pattern recognition” turns up thousands of entries on this topic.
To self-organize, life must have a discriminating algorithm that tells it what its own behalf is. Bacteria swim upstream in a glucose gradient. One need not confer consciousness on these single-celled organisms to acknowledge that this behavior manifests a rudimentary capacity for autonomy and pattern recognition, capacity to sense and respond to their environment. The A-PR cycle spirals from bacteria to humans as increasingly sophisticated pattern recognition is achieved.
There are many competing definitions of complexity. Ecosystems are multi-agent, complex systems where each autonomous agent is a pattern recognizer, interpreting signals in context. Overemphasis on EVO, survival of fittest algorithms, neglects the DEVO dynamic of self-organization in evolution. Zann Gill posits the A-PR Hypothesis (autonomy and pattern recognition), that life continually self-organizes, adapting toward increased functional complexity. Nature's processes manifest directionality without a goal state. Living systems operate as innovation networks, harnessing principles of collaborative intelligence: quorum-sensing in bacteria; ants as more than collective intelligence systems of pre-programmed robots, the theory of facilitated variation operating in our cells via weak linkage.
|
|
 |
|
Practical Applications. Computer scientists refer to granularity, the level of resolution at which pattern recognition occurs. With too much detail some patterns are obscured. With too little detail, other patterns cannot be recognized.
From micro level cellular mechanisms to macro level population genetics, evolution manifests a coordinated, multi-level Dyadic Model for innovation that next generation social networks could apply in decision support systems for eco-sustainability. Exponential acceleration of technology innovation extends the acceleration of biological evolution, posing challenges and opportunities. Competing hypotheses about the origin and evolution of life show nature’s adaptive mechanisms, evolvability, and the algorithmic implications of evo-devo debates, and also suggest a new collaborative computing paradigm, which can increase the effectiveness of human teams as “evolving, multi-agent ecosystems,” harnessing evolutionary principles to increase our collaborative intelligence.
Our current, conventional problem-solving model, based primarily upon “analysis of the facts,” is inappropriate for many of the problems we face today, which require a systematic (design) approach to synthesis and ways to acknowledge the role that human perception of the facts, and capacity for pattern recognition, plays in problem-solving.
|
|
 |
The distant (or not so distant) future vision of a system to support collaborative intelligence will be inspired by life-like systems with autonomy and capacity for pattern recognition (the A-PR Hypothesis), enabling them to navigate. Life-like capacity for pattern recognition and choice may exhibit what Babbage alluded to as a violation, an unpredictability to which the system must be able to adapt, and intelligence to respond to random unpredictable elements, accepting and adapting usable random variations. Babbage, the computer visionary, may have seen beyond the possibilities of today’s algorithmic computing to an era of intelligent computing with the unpredictabilities of life. If so, he anticipated that collaborative intelligence will require the capacity to accept and respond to unpredictability.
|
|
 |
Innovation (or Knowledge) Networks link participants, while maintaining their uniqueness and collaborative autonomy such that knowledge can evolve as networks grow, with potential for emergent, unpredictable patterns and innovative outcomes.
Problem mapping a priori, in contrast to information visualisation after-the-fact, generates visual frameworks, or “empty constructs” to structure the process of knowledge-gathering. Problem maps can evolve into navigable user interfaces. These open frameworks (partial patterns) tap the pattern recognition capabilities of users, serving as vehicles to order incoming information in process, and for use by participants during the problem-solving process. A classic example of a problem map is Dmitri Mendeleev’s Periodic Table of Elements, which prompted chemists to look for elements that appeared logically likely to exist, based upon the pattern of the Table.
|
|
 |
Situation architecture addresses the key factor of contextualization. Meaning is interpreted in context and may differ, not only with different interpreters, but in different contexts.
|
|
 |
Sustainability is the optimisation of tradeoffs to maximise environmental stewardship within the context of project planning priorities and, as defined by the Brundtland Commission, “the needs of the present without compromising the ability of future generations to meet their own needs.”
|
|
 |
Synergy is whole system behavior that cannot be predicted from the behavior of its parts.
Synergetics is the dynamics through which synergies are produced; both synergy and synergetics were key principles in the work of Buckminster Fuller.
|
|
 |
©2011 Zann Gill Please attribute, linking to this site.
Contact: webmaster at collaborative-intelligence. org
to top | home | links | compass
|